Research aims to create fairness in AI-assisted hiring systems
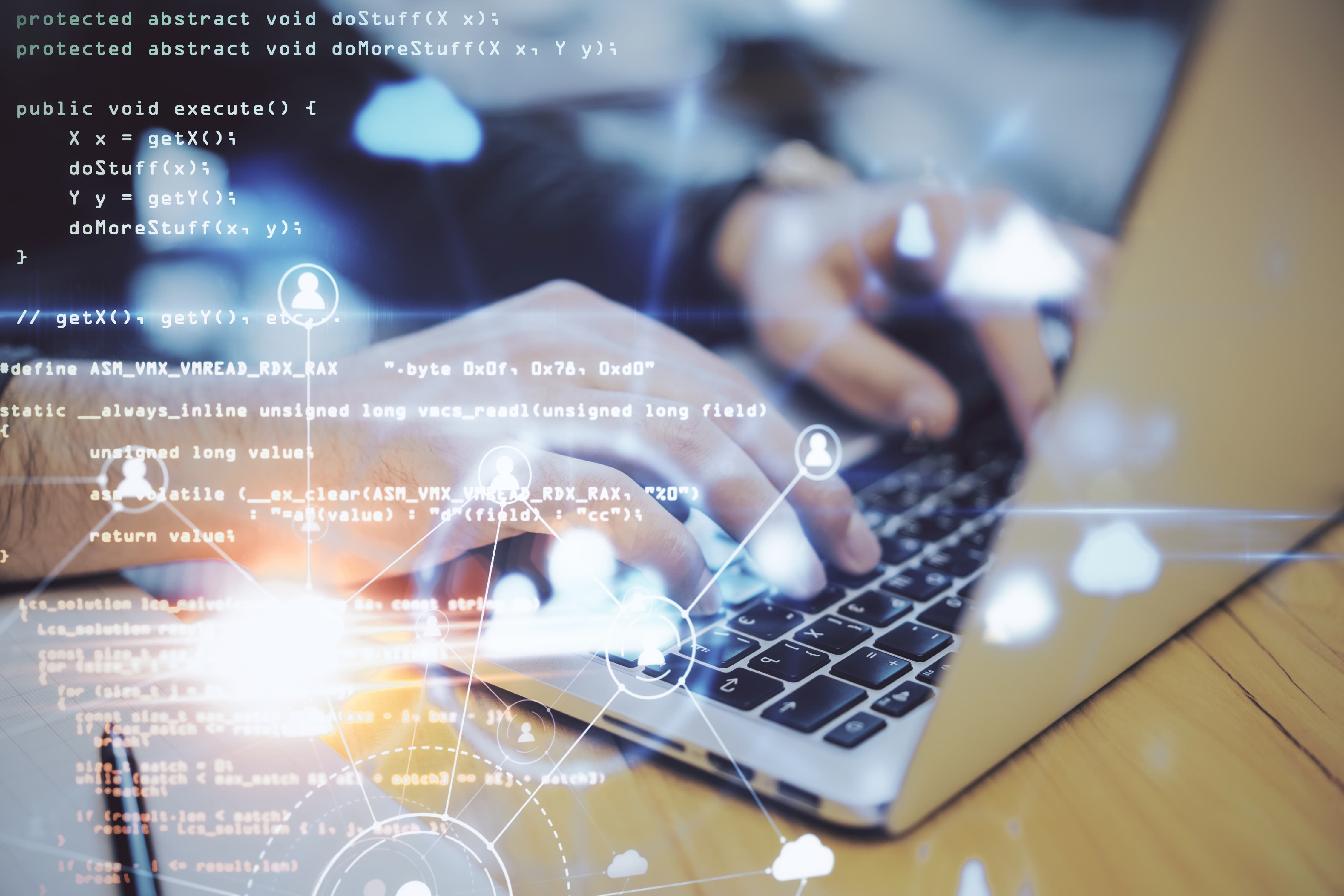
With an increasing number of employers using AI-assisted hiring systems to find ideal job candidates, researchers are investigating how to make these systems more trustworthy, transparent and fair. Credit: Shutterstock.
Welcome to From Florida, a podcast where you’ll learn how minds are connecting, great ideas are colliding and groundbreaking innovations become a reality because of the University of Florida.
Professor Mo Wang of the Warrington College of Business is in the early stages of a research project looking at how to design trustworthy, transparent and fair AI-assisted hiring systems – work funded by a grant of nearly $1 million from the National Science Foundation and Amazon. Wang talks about the project, why it is needed and what the team hopes to achieve in this episode of From Florida.
Transcript
Nicci Brown: Welcome to From Florida where you'll learn how minds are connecting, great ideas are colliding and groundbreaking innovation is becoming a reality because of the University of Florida. I'm your host, Nicci Brown.
Today we are joined by Professor Mo Wang, the Lanzillotti-McKethan Eminent Scholar in UF’s Warrington College of Business. Dr. Wang is in the early stages of a research project about fairness in AI hiring practices, an issue that is being widely discussed across our economy and workforce.
First, a little more about Dr. Wang. His work focuses on older worker employment and retirement, occupational health psychology, human resource management and quantitative methods. In addition to his role as a professor, Dr. Wang is the director of the Human Resource Research Center and chair of the Management Department. He is the founding editor of Work, Aging and Retirement, and has authored more than 200 scholarly publications. In addition to this, Dr. Wang is the incoming president of the Society for Industrial and Organizational Psychology for 2022-2023. Mo, thank you for joining us.
Mo Wang: Thank you. Thank you for having me.
Nicci Brown: You received a grant of nearly $1 million from the National Science Foundation and Amazon to study how to design trustworthy, transparent and fair AI systems to assist hiring decisions. Please tell us what you and your collaborators are looking at in your study.
Mo Wang: So, my research team, actually, we have computer scientists, we have information systems specialists, and then I am a psychologist. So what we are trying to do here is we are trying to improve the existing AI system for hiring to inject a social science perspective because so far a lot of the hiring decisions made by AI systems are designed by computer scientists. And often time their disciplinary training and also disciplinary tradition tend to pay less attention about the legal consequences and also the social evolutionary trends. So, we're trying to use this grant to inject this knowledge and then see whether we can build better system and also see whether we can actually eliminate the discrimination cases in the labor market.
Nicci Brown: So, building on that, much concern has been raised about hiring bias and AI is regarded as both a potential solution and a potential problem. So, let's start by talking about hiring bias. Can you tell us more about this problem?
Mo Wang: According to federal law, it is illegal to discriminate against a job applicant or an employee because of the person's race, color, religion, sex, national origin, age, disability status or genetic information. However, we know those kinds of discriminations happen on a day-to-day level. For example, in 2020 the EEOC, the Equal Employment Opportunity Commission, they actually received 22,000 charges on the basis of race-based discrimination. And a similar amount of charges were filed on the basis of sex-based discrimination and also disability-based discrimination. So, the discrimination in hiring is actually happening pretty frequently.
Nicci Brown: And let's talk, too, about the fact that not all of this is something that people are aware that they're doing. Some of this bias is actually implicit. They don't understand that they're being biased.
Mo Wang: Yeah, exactly. So, a big chunk of it is actually implicit bias, right? So, a lot of time people actually have discriminatory actions without even realizing, right? And also, of course, there are other more explicit bias, but more and more we see the implicit bias.
Nicci Brown: And AI is seen as a potential solution to this?
Mo Wang: Yes. So, AI has been viewed as a potential solution for two reasons. First, AI has been viewed as being more objective, so removing human bias, right? Because if the AI training algorithm is used, it can be blind to demographic information. So, from the surface you can see that all the algorithm does not consider like race, gender, age in evaluating the candidate. So, that's the first reason it's viewed as a solution.
The second reason is in the AI system, the algorithm can allow the desired fairness level to be specified as a parameter. And so, what that means is as a selection model, as a prediction model, so the AI system allows to do a complex mapping from predictors to decisions that optimize accuracy while satisfying the fairness constraint. Basically this is called in recent advancement, this is called fairness-aware AI system. So, it is viewed as helping with the fairness issues.
Nicci Brown: And yet there are still concerns about structural bias in algorithms, correct?
Mo Wang: Oh, yes. So, this is actually based on our recent research on the grant. So, the first thing is, although AI can be blind to demographic information for job candidates, it may still pick up other predictors that entail bias against the minority candidates. So, for example, if an AI system capitalizes on certain predictors that are prone to bias against the minority candidates, for example, criminal background, credit history or cognitive ability tests, well, it would generate a lower scores for those minority candidates although the demographic information is not explicitly in the model.
So, the second issue is actually related to the fairness-aware AI system I just talked about. So, the fairness-awareness AI system tends to select some candidates with high expected criterion and some others who look very much like minorities to satisfy the desired fairness level. However, when the AI system does that, it tends to create different predictive functions for different group of applicants. So, for example, the majority group and the minority group, their algorithm can be very different. So, that creates a differential treatment situation and we know that's not legal. So actually, although it's a fairness-aware AI system, by using the system itself, it creates a bias in its own form.
Nicci Brown: Yeah. So, it's by basically continuing on something that's biased to start with. So, it just extends that bias, say, with those kind of cognitive tests or whatever else it may be drawing on.
Mo Wang: Mm-hmm. Yeah, exactly.
Nicci Brown: So, there's no question that more employers, though, are relying on artificial intelligence to assist in their hiring decisions. So, what does this mean for job candidates? What can they do?
Mo Wang: So, actually this is a very good question. So, although more employers will rely on AI to assist in hiring decisions because it's automated, it's more efficient, the factors that make job candidates successful in jobs are relatively stable. It doesn't change very dramatically. So, in other words, so what the employers are looking for is still largely predictable. So, as a job candidate well, today what my employer wants from me would be largely stable like in, let's say, one or two years, right? So, a good AI hiring system should still pick up those factors in assessing job candidates. Therefore, when preparing for their job applications, job candidates need to understand what knowledge, skills and abilities or work styles that they should possess to be successful to perform the jobs they're applying for.
So, such understanding can help them better emphasize those qualifications in their job applications to enhance their chance to be selected. And one thing I want to mention may be helpful is actually the Department of Labor, the U.S. Department of Labor, hosts this online database called O*NET and it includes all kinds of information about jobs. So, basically when you develop your job applications, it would be good to search for that job in O*NET and find out what are the desirable levels of knowledge, skills, abilities and the work styles it require from you and emphasize that in your job application and that will enhance the chance.
Mo Wang: So, there are three things to consider when we design the AI system for hiring decisions. One is we need to minimize adverse impacts, which is we should have systems that generate the same selection ratio for majority and the minority groups. But at the same time to do that, we also need to minimize the predictive bias, which is what I was talking about. We need to avoid the differential treatment for different groups. So, they should share the same predictive function, because otherwise you are actually not using the same standard to hire people. But then at the same time, we also need to pay attention to the employer’s goal, right? So, employers want to maximize the fit between the job candidates and the job that they're hiring for. So, we also need to pay attention to that.
Our research actually shows of those three considerations, it's often easy to satisfy two of them and then to satisfy all three of them is quite difficult. So, our next step is to see how to optimize in this impossible triangle to create the best social value for the labor market. And then down the road, I think this may also have implication about the current employment law, because the employment law generally emphasizes the adverse impacts, right? So, most of system designers, they pay attention to this four-fifths rule, which is the minority selection ratio cannot be lower than 80% of the majority selection ratio. But our research shows that only paying attention to that is not enough, you also need to pay attention to the differential prediction for different groups. So, I think what we are going to do next step will have a lot of implication for guiding companies in designing those systems.
Nicci Brown: And when will the study be completed? Do you have a sense of that?
Mo Wang: So, the grant, it's a three-year grant, so we should wrap things up in 2024, but then we are also looking for other sources of funding maybe to continue this line of research. So, for example, so what we are looking at here are mainly a majority group versus minority group, but I also have research on aging, right? So, but when you look at people aging, so it's not majority versus minority because everyone ages. So, one day everyone become older. So, this kind of research will tackle different kind of discrimination issues. So, we're trying to also expand on that.
Nicci Brown: So, it sounds like this is going to be an ongoing and very interdisciplinary kind of work that you're doing. Mo, thank you so much for being our guest today. It's been a real pleasure.
Mo Wang: Oh, thank you so much for having me. I really appreciate the opportunity to explain my research. Thank you.
Nicci Brown: Listeners, thank you for joining us for an episode From Florida. I'm your host, Nicci Brown and I hope you'll return for our next story of innovation From Florida.